1. 3d dataset
3d를 표현하는 방법을 알았으니 데이터로 만들 수 있다면 3d를 이해하는 모형을 만들 수 있을 것
1) ShapeNet
51300개의 3d 모델이 55 category로 구성된 엄청난 large scale의 데이터
가상으로 사람들이 디자인한 high quality synthetic object
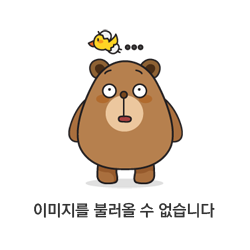
2) PartNet
ShapeNet의 개선 버전?으로 fine-grained dataset
하나의 3d object의 구성부분인 detail들이 annotation된 dataset
26671개의 3d model의 573585개의 part instance로 구성
part 뿐만 아니라 part의 구성요소도 색깔이 달라서 segmentation으로 굉장히 유용할 것
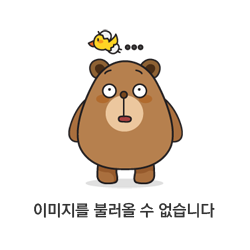
3) SceneNet
500만개 RGB이미지와 대응하는 depth map이 pair로 이루어진 data set
표현하는 이미지는 indoor(=실내의)이미지로 3d 모델로 시뮬레이션하여 synthetic하게 만들어 RGB와 depth로 rendering하여 만들었다?
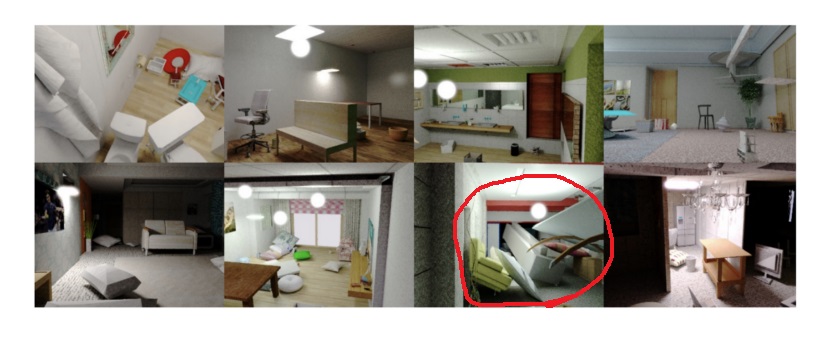
불가능하게 가구들이 존재하는 경우도 있는데 random하게 generation해서 그렇다
4) ScanNet
250만개의 view에서 구성한 RGB이미지와 depth map의 pair로 구성된 dataset
SceneNet과는 다르게 실제 1500개의 view 이미지에서 scan하여 만들었다
category도 있네
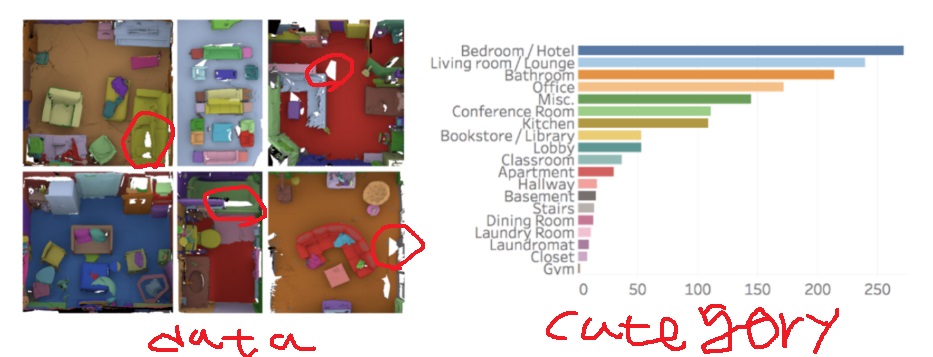
5) outdoor scene 3D dataset
보통은 무인자동차를 고려하여 만든 dataset
LiDAR data로 구성
LiDAR은 레이저를 쏴서 반사되어 돌아온 시간으로 물체의 위치를 추적하는 방법
보통은 3D bounding box로 label
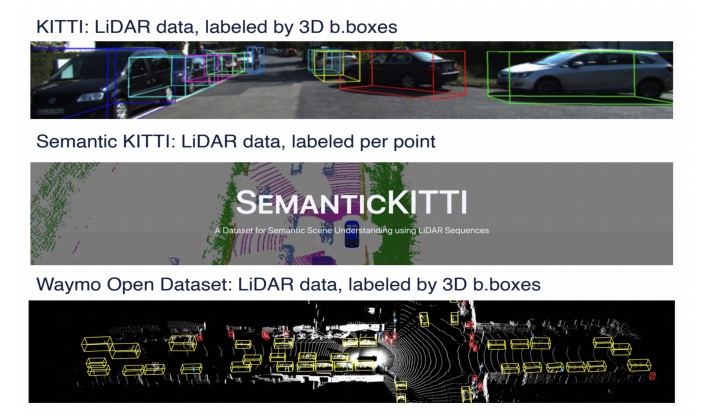
semantic KITTI같은 경우는 semantic segmentation을 위해 point로 label?
2. 3D task
3d data를 받아 3d용 neural network model에 넣어 label을 출력하는 recognition,
3d bounding box를 구하는 object detection부터 다양한 task들이 가능
1) 3D object recognition
2d image를 받아 CNN등에 넣어 label을 출력하듯이 3d도 마찬가지
3d object를 넣어 volumetric CNN같은 3D용 CNN에 넣어 하나의 label을 출력
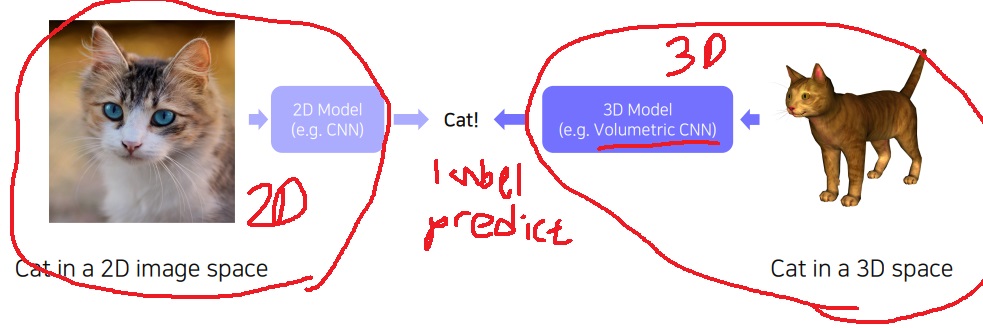
2) 3d object detection
3d object를 localization하는 문제
3d space에서 point cloud가 주어지면 3d bounding box를 구할 수도 있고 image에서 bounding box를 구할 수도 있다
무인 자동차 application에 주로 사용함
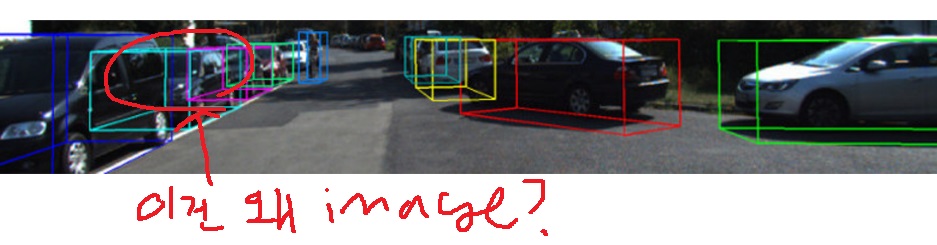
동그라미 부분이 왜 image에서 bounding box를 구한거지?라고 생각했는데
사실 위 전체 그림은 image에서 bounding box를 구한 예시가 아닐까?
point cloud(이게 그림에는 없으니까)에서 bounding box를 구한 예시는 아닌게 아닐까?
3) 3d semantic segmentation
3d mesh data나 neuroimaging에서 각 기능별 segmentation
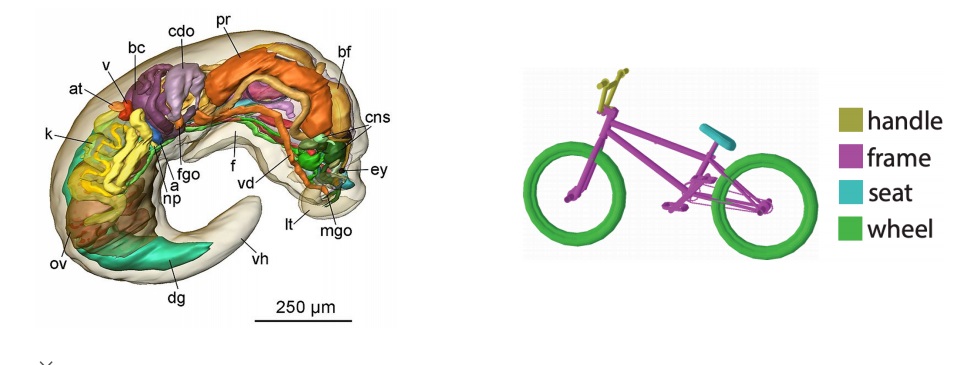
'딥러닝 > Computer Vision' 카테고리의 다른 글
visual and audio cross modal reasoning2 - speech separation, lip move generation (0) | 2024.09.10 |
---|---|
visual and audio cross modal reasoning1 - sound source localization (0) | 2024.09.09 |
3D 이해하기2 - 3D를 표현하는 방법 (0) | 2024.05.08 |
3D 이해하기 - 우리는 세상을 어떻게 관찰하는가? (0) | 2024.05.08 |
visual and audio matching modality - SoundNet (0) | 2024.05.02 |