1. Gradient Descent
미분가능한 함수의 반복적인 1차미분으로 국소적 최솟값(local minimum)을 찾는 과정
loss가 감소할 때 optimum이라 기대하는 지점이 존재하는 loss function이 존재할 것이라고 가정
최적을 찾고자 하는 parameter로 loss에 대해 편미분을 함
2. stochastic gradient descent
매 학습 스텝마다 random single sample 이용한 gradient 계산
random sample 1개만 사용하여 gradient를 계산함
가장 불안정하지만 가장 빠르게 계산 가능
3. mini batch gradient descent
매 학습 스텝마다 random subset of data sample을 이용한 gradient 계산
random sample의 일부분을 사용하여 gradient를 계산함
stochastic에 비해 느리지만 조금 덜 불안정해지니 가장 자주 사용함
4. batch gradient descent
일반적인 경사하강법으로 한번에 모든 데이터를 이용한 gradient 계산
너무 느리다는게 문제
그러나 제일 안정적
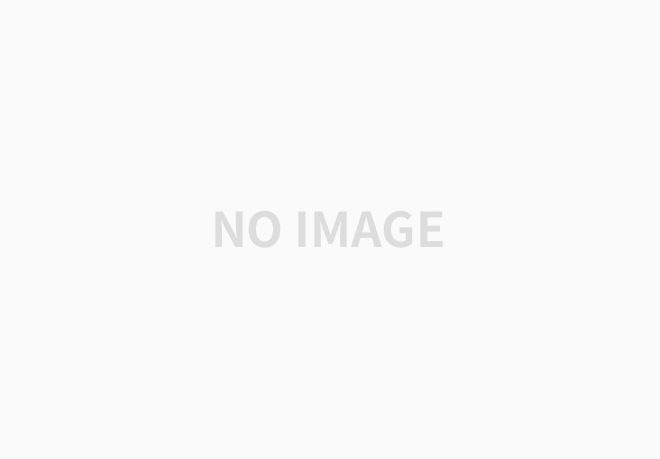
5. 참고
https://songminkee.github.io/studyblog/hands%20on%20machine%20learning/2020/05/15/4.html
4. 모델훈련 · Monch
15 May 2020 • Hands On Machine Learning 선형 회귀 일반적으로 선형 모델은 입력 특성의 가중치(weight) 합과 편향(bias)이라는 상수를 더해 예측을 만든다. \(\hat{y}=\theta_{0}+\theta_{1}x_{1}+\theta_{2}x_{2}+...+\theta_{
songminkee.github.io
'딥러닝 > 딥러닝 기초' 카테고리의 다른 글
경사하강법 알고리즘(gradient descent algorithm) (0) | 2021.12.30 |
---|---|
미분계수에 대하여 (0) | 2021.12.29 |
여러가지 regularization 기법 (0) | 2021.12.26 |
softmax function에 대한 진정한 의미 (0) | 2021.12.26 |
모델의 일반화능력(generalization performance) (0) | 2021.12.24 |