1. transfer learning
transfer learning은 특정한 task를 학습한 모델을 다른 원하는 task에 이용하는 모델링 방식을 말한다.
이전에 미리 학습한(pre-training) 모델이 가지고 있는 지식이 원하는 task에서 유용하게 활용될 수 있을 것이라는 기대가 있어서 그렇다.
실제로 사람도 이미 가지고 있는 지식<예를 들면 너의 통계학 지식>을 바탕으로
전혀 모르는 새로운 학습<예를 들면 처음하는 인공지능 이론 공부>에 경험이나 노하우 등을 유용하게 써먹잖아
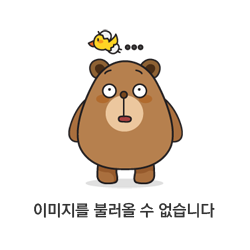
pre-train된 모델을 그대로 사용하거나 목적 task를 위한 작은 layer를 추가하여 학습하는 방식이 바로 transfer learning이다.
2. pre-training for up-stream task
pre-training 과정에서 수행하는 task를 특별히 up-stream task라고 부른다.
대표적으로 다음 단어를 맞추는 학습<gpt-1이 이 방식을 사용했다>, mask된 단어를 맞추는 학습이 있다.
up-stream task를 수행하는 학습 과정이 바로 model의 pre-train과정이다.
pre-train에서 사용하는 data1은 label이 없는 unsupervised data이다.
모델 학습에 대량의 데이터를 사용할 수 있다는 장점이 있다.
Unsupervised pre-training is a special case of semi-supervised learning where the goal is to find a good initialization point instead of modifying the supervised learning objective.
unsupervised pre-training은 진짜 목표로 하고자하는 supervised learning을 위한 좋은 가중치 초기화방법으로도 볼 수 있다.
3. transfer learning for down-stream task
down-stream task란 pre-train된 모델을 가지고 진정으로 수행하고자하는 목적에 해당하는 task
pre-train 모델을 transfer learning로 학습한다. 이렇게 pre-train을 한 이유는 down-stream task를 더욱 잘하기 위해서다.
대표적으로 question and answering, document classification 등이 있다.
3-1) fine-tuning
down-stream task를 위한 data를 사용하는 방식에 따라 구분지을 수 있다. 그 data를 모두 사용하여 학습하는 방식이 fine-tuning이다.
pre-train으로 좋게 초기화된 가중치를 가진 모델에서
down-stream task를 위한 supervised data를 모두 학습하여 미세하게 가중치를 조정한다는 의미에서 fine-tuning이라는 이름이 붙었다.
3-2) zero-shot learning
data를 하나도 사용하지 않고 pre-train model을 바로 down-stream task에 실험해보는 방식이다.
pre-train으로 얻은 지식으로 바로 시험보는 거다.
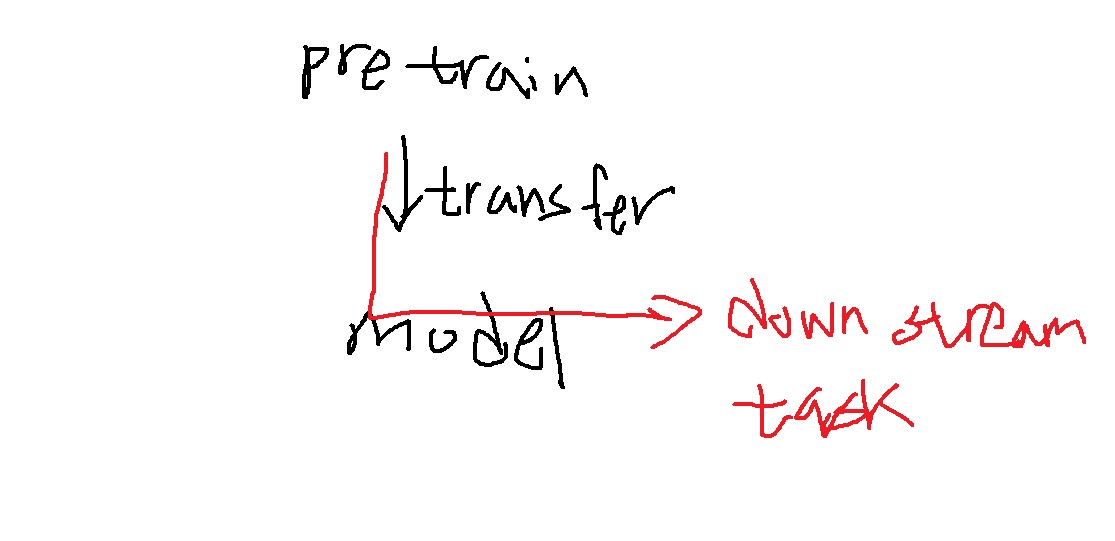
3-3) one-shot learning
down-stream task를 위한 데이터를 하나만 사용하여 pre-train model을 학습시키고 실험해본다.
3-4) few-shot learning
down-stream task를 위한 데이터를 일부만 사용하여 pre-train model을 학습시키고 실험해본다.
4. 예시로 이해하는 GPT-1의 transfer learning
다양한 text data를 모두 사전에 학습한 GPT-1을 이용해 여러 주제를 가지는 document의 category를 classify하는 문제를 풀어야한다면?
기본적으로 제공했던??? GPT-1의 fine-tune모형인 sentiment classification은 binary classification이어서 document classification같은 categorical classification에 사용하기는 어렵다.
그래서 pre-train한 self-attention block(transfer model)은 그대로 두고 sentiment classification에 사용한 output layer만 제거한다.
그리고 self-attention block뒤에 document classification을 위한 output layer를 새로 만드는 것이다.
이 새로만든 output layer의 가중치를 적절하게 초기화하고 supervised document data를 이용해 새로운 학습을 진행하는 것이다.
이미 가중치가 잘 선택된 pre-trained self-attention block에는 적은 learning rate를 주어 가중치가 미세하게 조정되도록 한다.
random initialization된 새로운 document classification output layer의 가중치를 적절하게 학습하는 것이 목적이다.
이 과정이 바로 fine-tuning과정이면서 GPT-1의 transfer learning이다.

오랜만에 그림 보니까 진짜 못그리긴했네;;
기존에 있는 output layer를 먼저 제거(sentiment classify를 위한 layer를 제거)
목적에 맞는 down stream task를 위한 output layer(document classify)를 붙여버리고 가중치를 초기화(random initialization)
새로운 데이터로(new data)부터 학습을 진행, 정답을 아니까 그 정답이 나오도록 softmax loss를 최소화하는 방식으로 가중치를 학습
pre-train된 self attention block의 가중치에는 적은 learning rate를 주어 미세하게 변화되도록 한다.(weight fine tune)
5. 참고
https://ratsgo.github.io/nlpbook/docs/introduction/transfer/
Transfer Learning
pratical tips for Natural Language Processing
ratsgo.github.io
https://cdn.openai.com/research-covers/language-unsupervised/language_understanding_paper.pdf
'딥러닝 > NLP' 카테고리의 다른 글
현대 NLP 모델의 근간이 되는 BERT의 기본적인 특징 (0) | 2022.10.24 |
---|---|
괴물 언어모델 GPT-1에서 더 강력해진 GPT-2 파헤치기 (0) | 2022.10.21 |
NLP의 최신 트렌드 - GPT-1 파헤치기 (0) | 2022.06.28 |
transformer의 마지막 encoder-decoder multi head attention (0) | 2022.05.18 |
transformer decoder에 사용된 masked self attention에 대해 알아보고 구현하기 (0) | 2022.05.18 |